1.
- Open-source: Free to use and distribute.
- Powerful: Offers a wide range of statistical functions and packages.
- Flexible: Can be customized to fit specific needs.
- Learning curve: May have a steeper learning curve for beginners.
2. Python:- Versatile: Can be used for general-purpose programming and statistical analysis.
- Popular: Has a large community and many libraries for data analysis (eg, NumPy, pandas, SciPy, Statsmodels).
- Ease of use: Relatively easy to learn for beginners.
3. SPSS:- Commercial software: Requires a license.
- User-friendly: Intuitive interface and pre-built analyses.
- Powerful: Offers a wide range of statistical features.
4. SAS:- Commercial software: Requires a license.
- Robust: Known for its reliability and scalability.
- Widely used: Popular in industries like healthcare and finance.
5. MATLAB:- Commercial software: Requires a license.
- Matrix-oriented: Designed for numerical computing and data analysis.
- Visualization: Powerful tools for creating visualizations.
6. Minitab:- Commercial software: Requires a license.
- User-friendly: Easy to learn and use.
- Specialized: Focused on statistical analysis and quality improvement.
7. Stata:
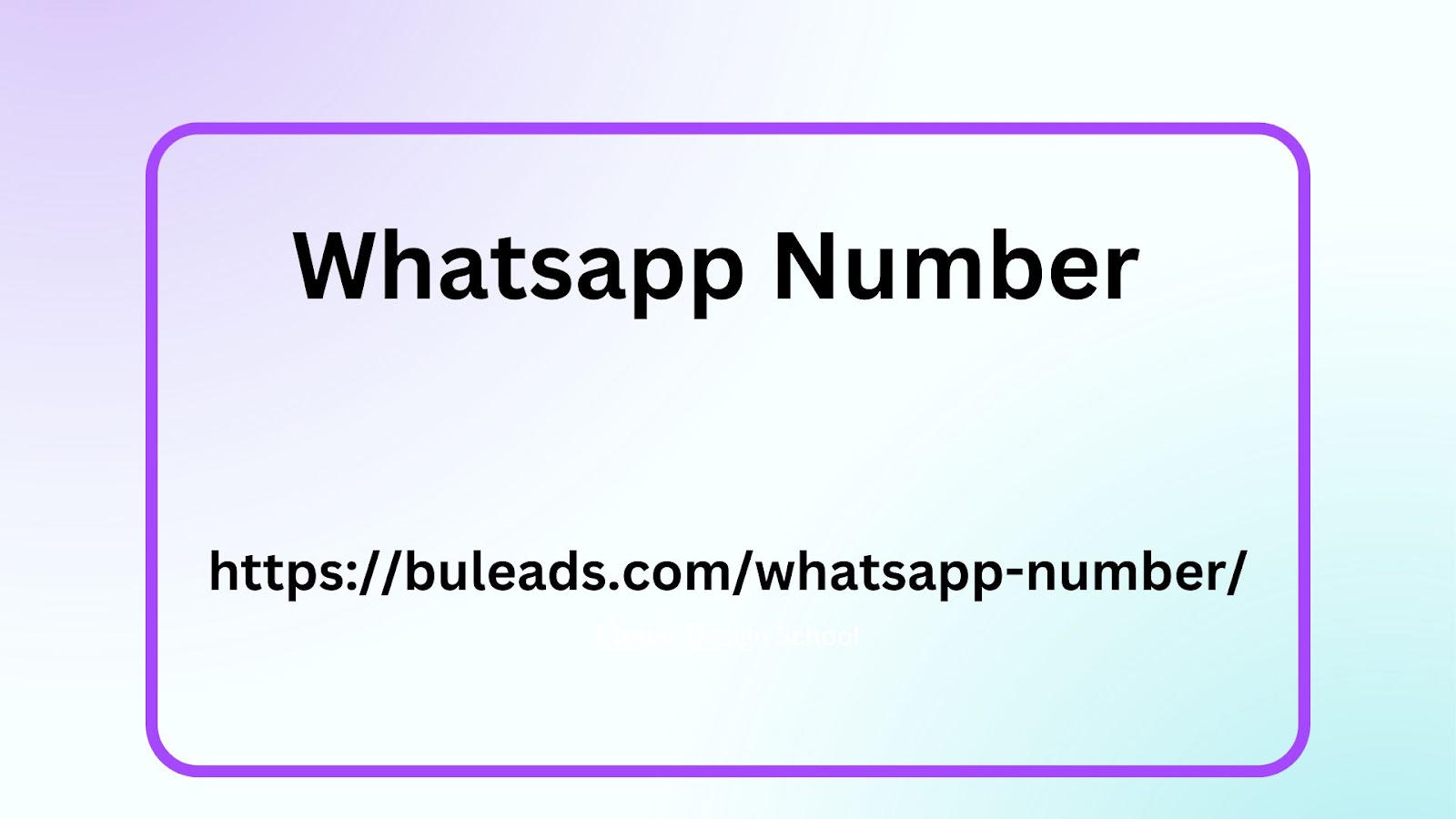
- Commercial software: Requires a license.
- Econometrics: Strong capabilities for econometric analysis.
- Data management: Efficient data management tools.
Factors to consider when choosing software: - Cost: Free vs. commercial software.
- Ease of use: How easy is the software to learn and use?
- Features: Does the software offer the specific features you need?
- Community: Is there a large and active community of users?
- Integration: Can the software be integrated with other tools you use?
By carefully evaluating these factors, you can select the data statistical analysis software that best suits your needs. |