|
Advantages and Disadvantages of SVM Advantages: Strong generalization ability Effective in high-dimensional space Applicable to a variety of kernel functions Performs well on small sample data sets Disadvantages: Sensitive to parameter adjustment Long training time on large data sets Sensitive to noisy data Comparison of SVM with other algorithms Algorithm Advantages Disadvantages SVM Strong generalization ability.
applicable to high-dimensional data Email List Sensitive to parameters, long training time Decision tree Easy to understand, strong interpretability Easy to overfit Random forest High accuracy , strong anti-overfitting ability Complex model, difficult to explain Neural network Applicable to complex problems, strong learning ability Requires a lot of data, long training time Export to Sheets Summary SVM is a powerful machine learning algorithm with a wide range of applications in the field of data mining.
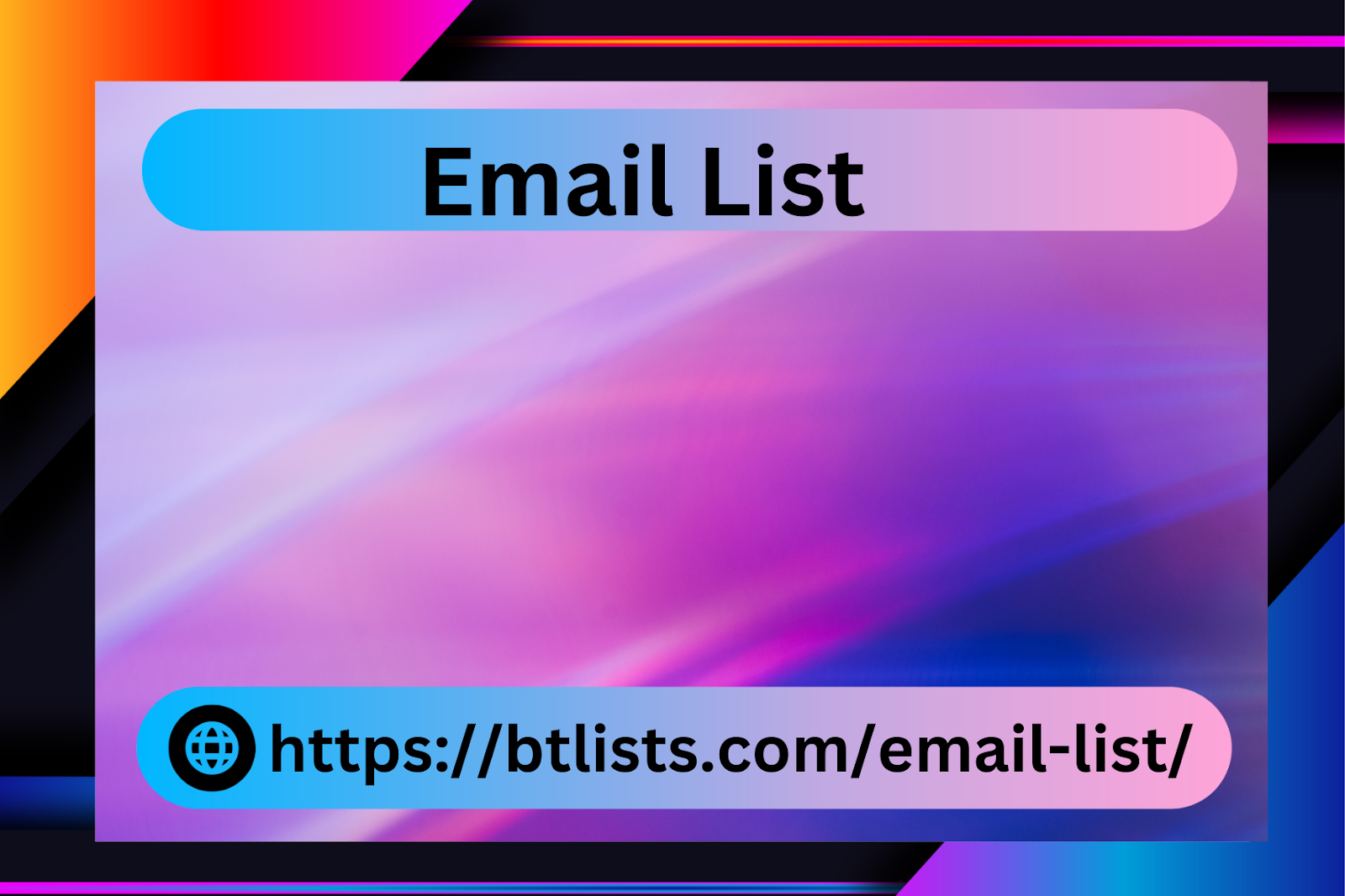
It performs well in processing high-dimensional data, small sample data, and nonlinear problems. However, SVM also has some disadvantages, such as sensitivity to parameters and long training time. When choosing an algorithm, it is necessary to consider various factors based on the characteristics of the specific problem and data set. Further learning If you want to learn more about SVM, you can refer to the following: Mathematical foundation: linear algebra, probability theory, optimization theory Machine learning foundation: supervised learning, unsupervised learning Mathematical principle of SVM: Lagrange duality, kernel function, etc.
|
|